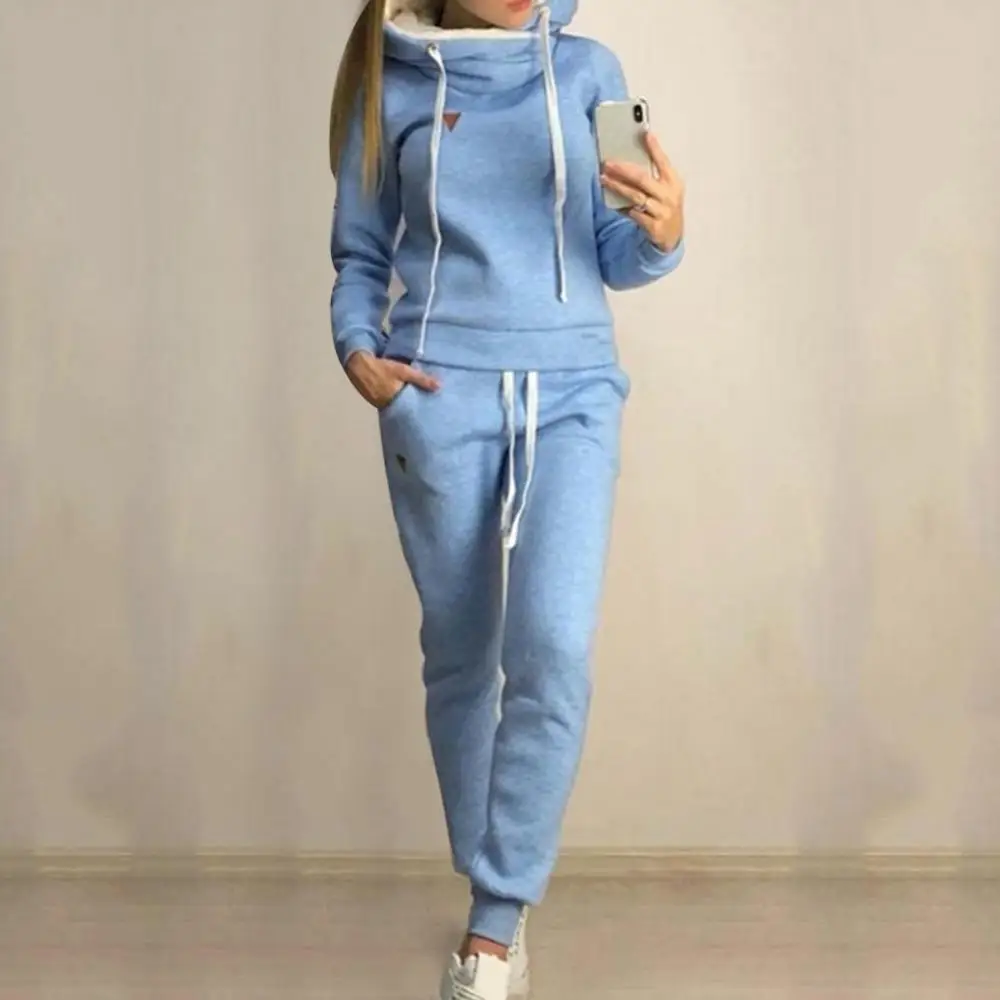
Vásárlás Melegítőben egyszínű nők 2db szabadidőruha melegítő fleece bélelt kapucnis felső nadrág szett - Futó Szett < Aprive.io
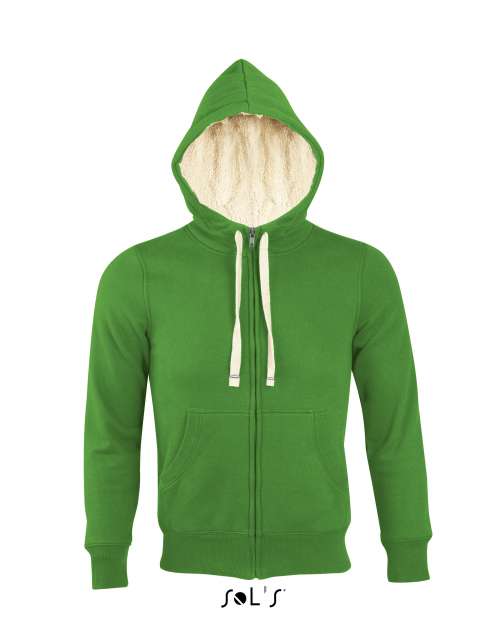
Márkás pólók, szabadidőruhák | SOL'S SHERPA (SO00584) uniszex pulóver poliészter/pamut oldalvarrott karcsúsított zsebes végig zipzáras kapucnis bélelt, Rügyzöld/Bud Green színben, méret: L | M3munkaruha munkaruházati, munkavédelmi eszköz és tűzoltó ...
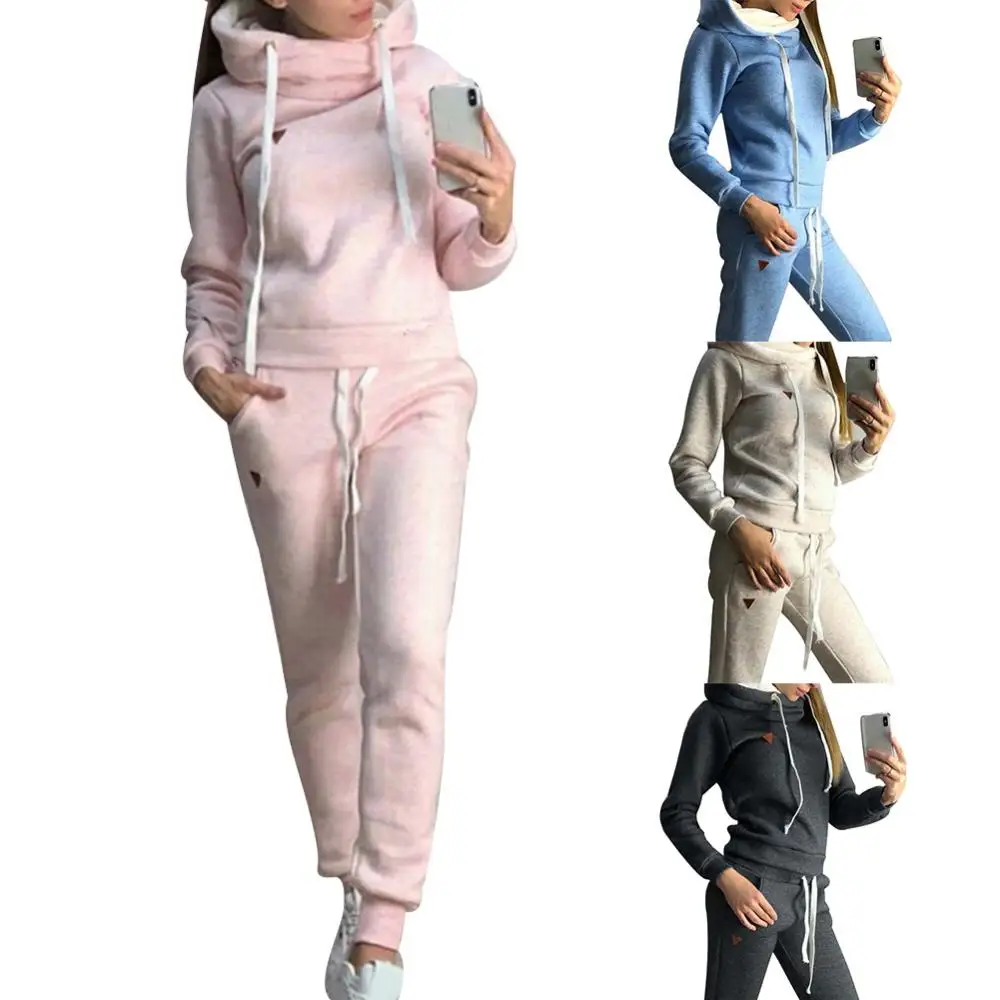
Vásárlás Melegítőben egyszínű nők 2db szabadidőruha melegítő fleece bélelt kapucnis felső nadrág szett - Futó Szett < Aprive.io
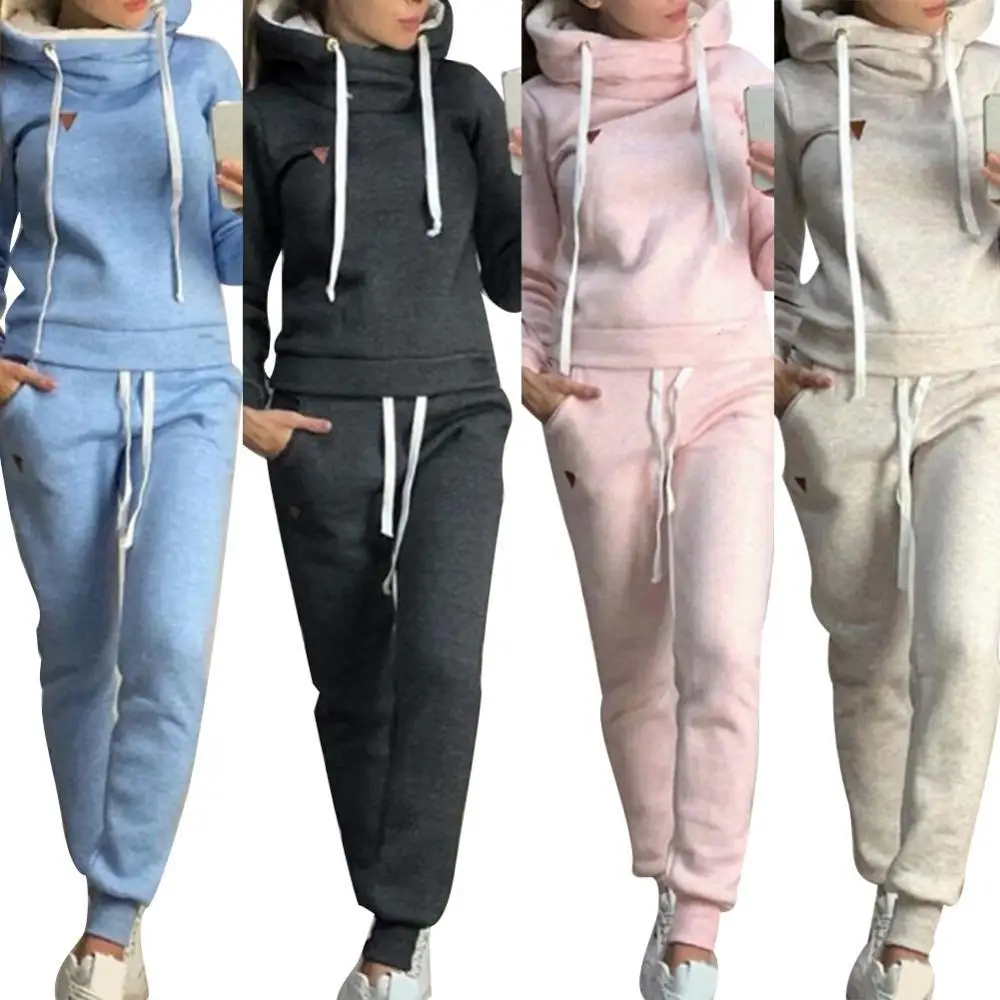
Vásárlás Melegítőben egyszínű nők 2db szabadidőruha melegítő fleece bélelt kapucnis felső nadrág szett - Futó Szett < Aprive.io
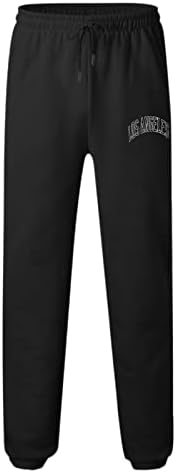
Férfi 2 Db Öltöny Alkalmi Relaxed Melegítő Divat Kapucnis Sportos Aktív Melegítő - Lignesdereperes.com
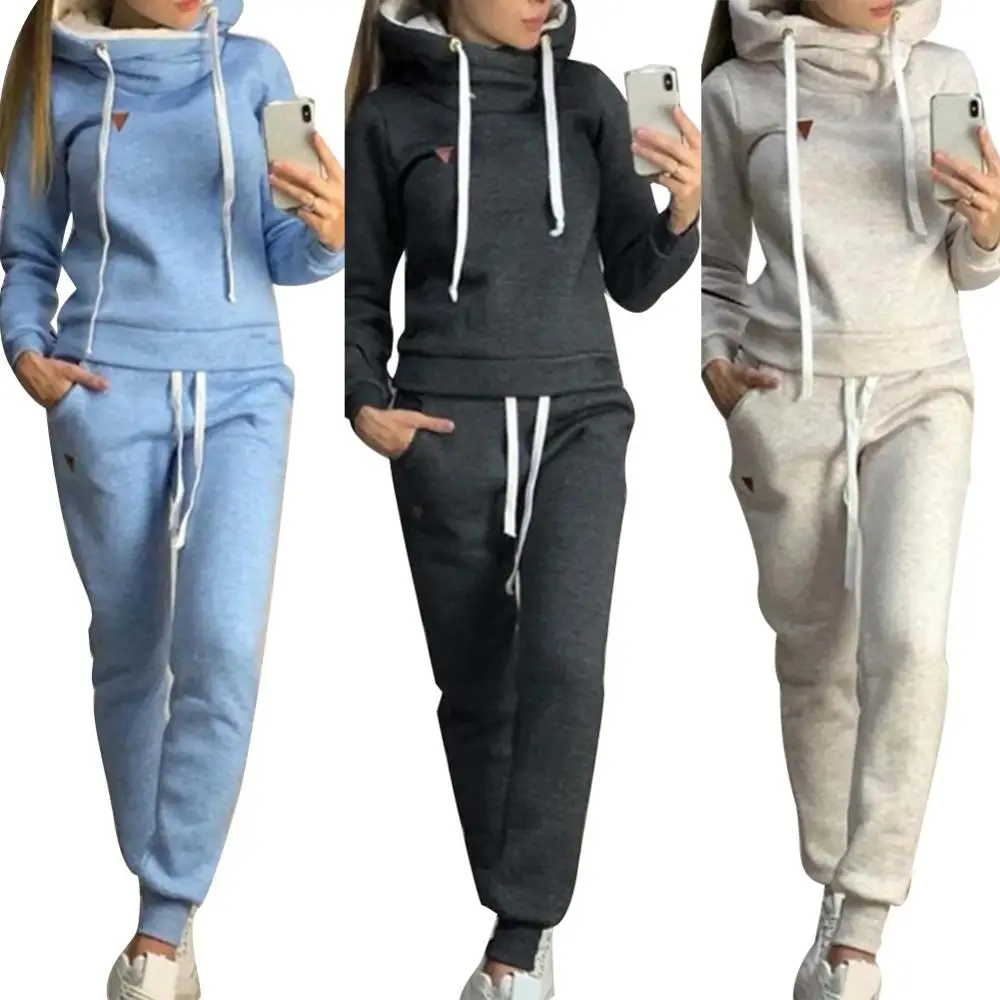